Customer Centricity in the Airline Industry
Competing merely in terms of market share may have become inadequate for most major traditional airlines to sustain a profitable business in the long run. The entry of new competitors is reshaping the market distribution vastly against the traditional players. Those new challengers snatch new market share either with new business models and slightly different products—e.g., low-cost carriers that appeal to the budget sensitive customers, who constitute a substantial portion of the market (Dresner et al., 1996; Bilotkach et al., 2011; Budd et al., 2014) or with competition from countries with lower operational costs (and/or benefiting from unfair subsidy schemes, due to a lack of regulation in this industry (Dresner et al., 2015; Tretheway & Andriulaitis, 2015).
With a product-focused approach, profitability is tied to market share (leveraging the economy of scale). By contrast, with a customer-focused approach, which consists in influencing customers so that they prefer a particular firm (or brand) over its competitors, profitability arises from building loyalty (multiple transactions) that reposes on delivering bespoke solutions to a selected group of customers (who are willing to pay a premium for tailored products) (Keiningham et al., 2012). Similarly to the product centric paradigm (i.e., market share competition), a customer-centric initiative achieves profitability via customer acquisition, retention and development, but in a much smarter way. The required expertise is not only about products but about relationships as well.
Although traditional airlines are perceived as rather conservative, Harteveldt (The Future Of Airline Distribution - A Look Ahead To 2017, 2012) recalls that the early stage of eCommerce emerged from the airline industry back in the 1950s—see the Sabre system (Wikipedia, 2015). He wrote (Harteveldt, 2012, p. 3):
Although airlines don’t fly the same planes in 2012 that they did in the 1950s, 1960s, or 1970s, parts of the industry’s business processes, business models, and technology infrastructure date back to those eras.
Exploring new potential means of generating long-term profitability has become essential for traditional airlines (Pilz & Dyerfox, 2011). The information era that is in its way of superseding (in terms of business and lifestyle impact) the technological revolution of the last century is bringing about new tools that put a genuine customer centric approach within reach.
A customer-centric approach requires knowing in depth the (prospective) customers in order to precisely estimate their lifetime value. The extreme-fast-pace accumulation of both structured and unstructured data together with the recent progress in machine learning techniques are demonstrating mind-blowing results. Artificial intelligence of the beginning of this 21st century is already successfully delivering beyond its promises, and this is only the start of the exponentially ascending curve (to illustrate this fact, notable figures such as Stephen Hawking, Bill Gates or Elon Musk, among others, have recently expressed some grave concerns about the threat that such a technology could pose on human kind–a quick search using Google News yields many results on that topic). kurzweil (IT Growth and Global Change: A Conversation with Ray Kurzweil, 2011) famously said, “information technology’s growing exponentially and our intuition about the future is not exponential, it’s linear.” Those intelligent systems are not only confined in research labs, they are invading the industry as well (Power, 2015).
Other industries have already harnessed those technologies to successfully implement customer centric approaches. What about traditional airlines that might still have the resources to make the jump?
The Value-Discipline Model
Treacy and Wiersema (The Discipline of Market Leaders: Choose Your Customers, Narrow Your Focus, Dominate Your Market, 1997) introduced the Value-Discipline Model that distinguishes three value disciplines:
- Operating excellence,
- performance superiority and
- customer intimacy.
Treacy and Wiersema (“Customer Intimacy and Other Value Disciplines,” 1993, para. 4) claim that for a firm to be competitive, it must “become champions in one of these disciplines while meeting industry standards in the other two.” The model assumes that a customer picks the company that excels in the one discipline she/he values the most. Figure 1 illustrates the three values on a three-axis representation that could be used to visually compare different firms.
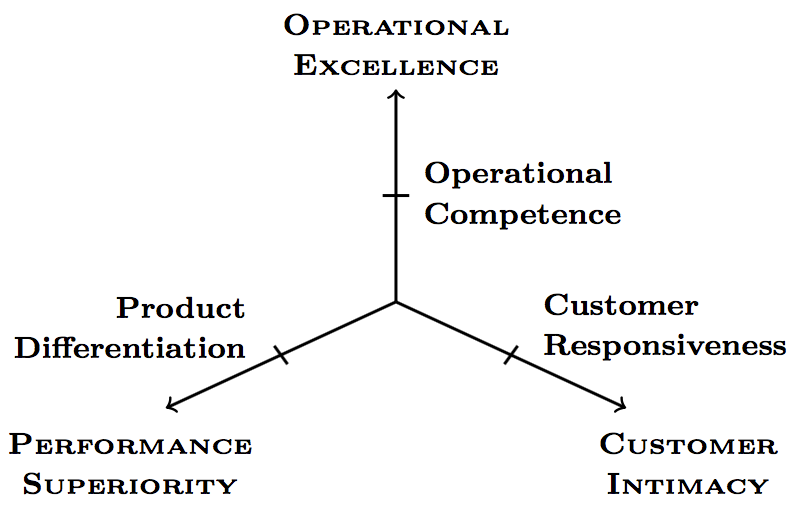
The authors (“Customer Intimacy and Other Value Disciplines,” 1993, para. 6) suggest that it is not possible for a firm to master all the three disciplines. This claim is rationalized by the fact that in order to reach excellence in one dimension, “the leaders have aligned their entire operating model—that is, the company’s culture, business processes, management systems, and computer platforms—to serve one value discipline,” leaving enough resources and degree-of-freedom only to deliver fair value in the two other axes. They, however, describe a few instances where “maverick companies,” as they call them, have reached mastery in two disciplines.
Major traditional airlines have lost the fight for operational excellence against the low-cost carriers (Smyth & Pearce, 2006; Moosberger & Pearce, 2007; Barbot et al., 2008). Acquiring mastery of operational excellence to rival that of the low-cost carriers may not be realistic—e.g., many of those airlines are unionized, leaving only little flexibility for implementing drastic changes required for such a crusade (Blyton et al., 2001; Hätty & Hollmeier, 2003). In this context, it appears wise to continue to strive to increase their operational capabilities, so as to maintain a fair value in this axis, while developing excellence in one of the two other value disciplines. But which one? The performance superiority or the customer intimacy? The latter seems the wise choice. Why? First, the core business of most airlines is mobility. Product excellence would therefore consist in enhancing mobility to the point of outperforming competitors. We can safely conjecture that a teleportation machine is not in the horizon. Mobility can be improved (from an airline perspective) by easing the hassle of unexpected events, e.g., by offering affordable flexibility. Alternatively, airlines might expand their services beyond the airports (and thus, e.g., release the stress engendered by the fear of missing a connection, such as a train, due to a delay at the arrival airport—as the expenses incurred would then presumably be borne by the airline).
A passenger wants to go safely and in a predictable way (i.e., on schedule) from A to B with her/his belongings. Entertainment, food and comfort, while being of great significance, are not the focus (and particularly on short haul where even wealthy passengers may not pay much attention to the quality of the experience). Regarding those aspects, airlines are practicing a second-order price discrimination to offer fair value to a large panel of passengers, through the different classes (product differentiation to match willingness to pay). Although it remains essential to continuously improve the services, it sounds quite challenging to truly achieve performance superiority in the highly-regulated airline business.
To pursue mastery in customer intimacy, on the other hand, appears both within reach (though challenging) and of potentially great benefits. Besides, there seems to be a demand, judging from the analysis conducted by Google and mentioned by Harteveldt (The Future Of Airline Distribution - A Look Ahead To 2017, 2012, p. 4): “Google analysis shows that travellers shop on 22 websites before booking.” Furthermore, a study conducted by IBM revealed that the recent improvements in operational capabilities came at the expense of customers’ satisfaction, a context prone to a boomerang effect (Pilz & Dyerfox, 2011). A decline in passengers’ satisfaction means a setback on the customer intimacy line; a tendency that might be urgent to reverse.
In this industry, what is really peculiar is that the perceived operational excellence by passengers probably remains below the fair-value line—see, e.g., the CODA’s report (Delays to Air Transport in Europe - Annual 2013, 2013) or the work of Sherry (“A Method for Quantifying Travel Productivity for Corporate Travel Managers,” 2015). Many flights are either canceled or significantly delayed on a daily basis due to factors that operators cannot really influence, such as the weather conditions or the congestion of certain popular airports. The impact on customers’ perception is even much worse when the disruption is due to a strike (which is, unfortunately, all too frequent an event that plagues traditional airlines—and which can, in some instances, be triggered by some measures taken to further enhance the operational competence). The IBM report (Pilz & Dyerfox, 2011) mentions that in 2010 the American Customer Satisfaction Index (theacsi.org) ranked the air travel industry 42nd out of 44 in customer satisfaction.
Customer Centricity
The term customer centricity seems to be shrouded in terminological ambiguity. The confusion arises from failing to recognize the distinction between being customer friendly (or customer focused) and being customer centric. The scope of the latter fully encompasses the former concept, but is broader. Fader (Customer Centricity, 2012, chap. 2) defines customer centricity as follows:
Customer centricity is a strategy that aligns a company’s development/delivery of its products/services around the current and future needs of a select set of customers in order to maximize their long-term financial value to the firm.
This definition is rather abstract, what does it entail in practice? One crucial point is “select set of customers.”
The concept of selecting customers is not new, for this is a key point in building a strong brand. The segmentation, targeting and positioning approach (known by its acronym STP) is de facto a process that selects a group of customers. However, this is a passive selection, in the sense that customers are not treated differently depending on their estimated future value. This is in sharp contrast with customer centricity, in which the targeted customers are actively favored over the other customers.
In the airline industry, the customers lock-in mechanism is typically implemented via the mileage system (often referred to as a frequent flyer program, and aims at preventing customers from switching to competitors). It is designed to reward the customers that have used the service the most in a short past period (and the reward is proportional to the utilization). Although this may be subtle, here all the customers are indifferently treated. Any customer is entitled to the reward; in other words, anybody can purchase miles and get the associated benefits. Besides, the reward is based on the past profits, not future value. Are those passengers with many accumulated miles profitable on the long-run? It is basically impossible to decide only from their membership status. This is a very efficient lock-in strategy but does not directly align with a customer-centric approach (this can be a significant part of it, though). Schmidt and Rosenberg (How Google Works, 2014, chap. 2) advocate against customer lock-in, presumably because it can somehow be perceived as coercive (it might be worth mentioning, though, that Google is vastly superior to the competition in many measures, which can without doubt ease the development of such a belief by its CEO). Nevertheless, mileage programs are in general well appreciated by passengers, and have become an essential point-of-parity between airlines. A true customer centricity endeavor would probably redefine the mechanism of awarding miles to not only retain customers, but to acquire new customers as well.
One crucial principle of customer centricity is to evaluate the value of a customer. Potentially profitable customers must be distinguished from less profitable ones. The focus is set on customer lifetime value (i.e., present and future) rather than past observed profits. The aim is to acquire the customers with the highest lifetime value before a competitor does (selective customer acquisition), and invest in sustaining the relationship over the full period of time (customer retention and development). The investment should be coherent with the estimated value of the customer (the so-called lifetime value); in financial terms, the net present value (NPV) must be kept positive. From a pure financial perspective, it is reasonable and wise to invest capital today for acquiring a specific customer to the extent that the NPV of that customer remains looking good.
Many reports agree on the necessity to take measures in order to improve customer friendliness, which is essential to drive long-term loyalty (Pilz & Dyerfox, 2011). But very few, if any, discuss the estimation of customers’ lifetime value (required to achieve true customer centricity). Those reports seem to suggest that all customers are equal. An assumption that is not in line with customer centricity. Fader of the well-regarded Wharton Business School, author of Customer Centricity (Fader, 2012), gave an example during a video session of Wharton’s students resigning from their current role to undertake a two-year MBA program. It is likely that a large share of those students enjoyed privileges through their airline membership status acquired via numerous business travels prior to their enrollment. During their studies, however, those privileges will vanish, and after graduation, those newly graduates have to start from scratch in mileage terms. Yet, it is plausible that among them many have a huge lifetime value to an airline. It might be then judicious for airlines to offer special treatment to their existing members that enroll in a top business school program. It must be borne in mind, though, that this example draws a conclusion based on intuition. An objective method based on data should be used instead to derive a proper conclusion. Schmidt and Rosenberg (How Google Works, 2014, chap. 2) recall that the CEO of Netscape Jim Barksdale once said: “If we have data, let’s look at data. If all we have are opinions, let’s go with mine.”
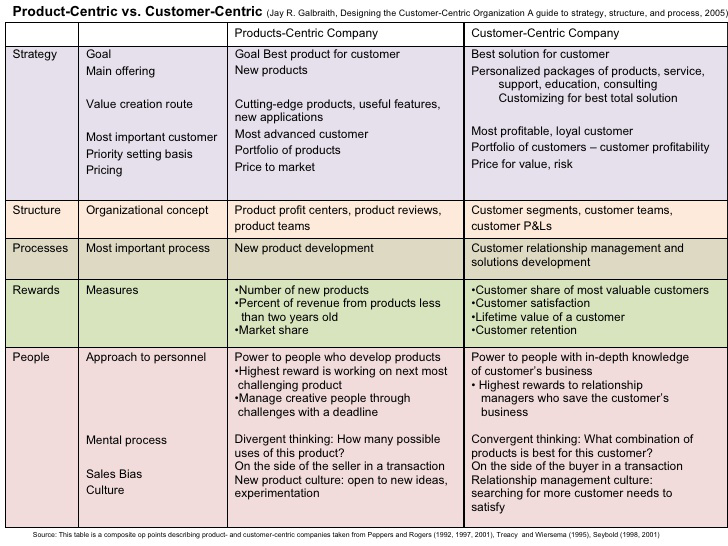
A Herculean effort is mandatory to tackle the switch from product centricity to customer centricity. The challenge is at least two-fold: managerial and technological (Brown et al., 2011). Regarding the former, Fader (Fader, 2012) stressed that:
Customer centricity requires the company to be willing and able to change its organizational design, performance metrics, and employee/distributor incentive structures to focus on this long-run value creation/delivery process.
Figure 2 depicts a table that summarizes the difference between a product centric organization and a customer centric one (source and credit are specified on the figure).
Concerning the latter, there probably exist several solutions; the current trend suggests that machine learning fueled with big data might have a high potential to deliver adequate outcomes—see, e.g., (Spiegel et al., 2013; Duhigg, 2012).
Big Data and Machine Learning
The Technology
Machine learning technology has gained unprecedented popularity among scholars and industries in the recent years. To get a complete snapshot of fundamental machine learning techniques I recommend the book Pattern Recognition and Machine Learning authored by Bishop (Pattern Recognition and Machine Learning, 2006). This book has now become quite old, but the maths behind the main principles have not changed much. Neural networks—recently called deep learning—are again believed to be promised to a bright future (Wikipedia, 2015). Demand for experts in such techniques has simply skyrocketed in the Silicon Valley. All the major actors in the field are investing big, very big: Google, Apple, Amazon, Facebook, Baidu, Microsoft, IBM to cite only the prominent ones.
“Big Data” has a semantic problem, and is far more ambiguous than customer centricity. Scholars, engineers or corporate executives might use the term to describe different concepts (De Mauro et al., 2015). IBM has set up a dedicated web page on the subject in which one will fail to find a formal definition; instead the following description is asserted:
Big data is being generated by everything around us at all times. Every digital process and social media exchange produces it. Systems, sensors and mobile devices transmit it. Big data is arriving from multiple sources at an alarming velocity, volume and variety. To extract meaningful value from big data, you need optimal processing power, analytics capabilities and skills.
De Mauro et al. attempted to extract a consensual definition from an extensive study of the literature (De Mauro et al., 2015). They conclude that big data refers to
information assets characterized by such a High Volume, Velocity and Variety to require specific Technology and Analytical Methods for its transformation into Value.
As its name would have let us guess correctly, the general consensus dictates that big data involves a data set (i) that is large and can be astronomically large (posing new challenges for both storage and processing), and (ii) that is continuously expanding.
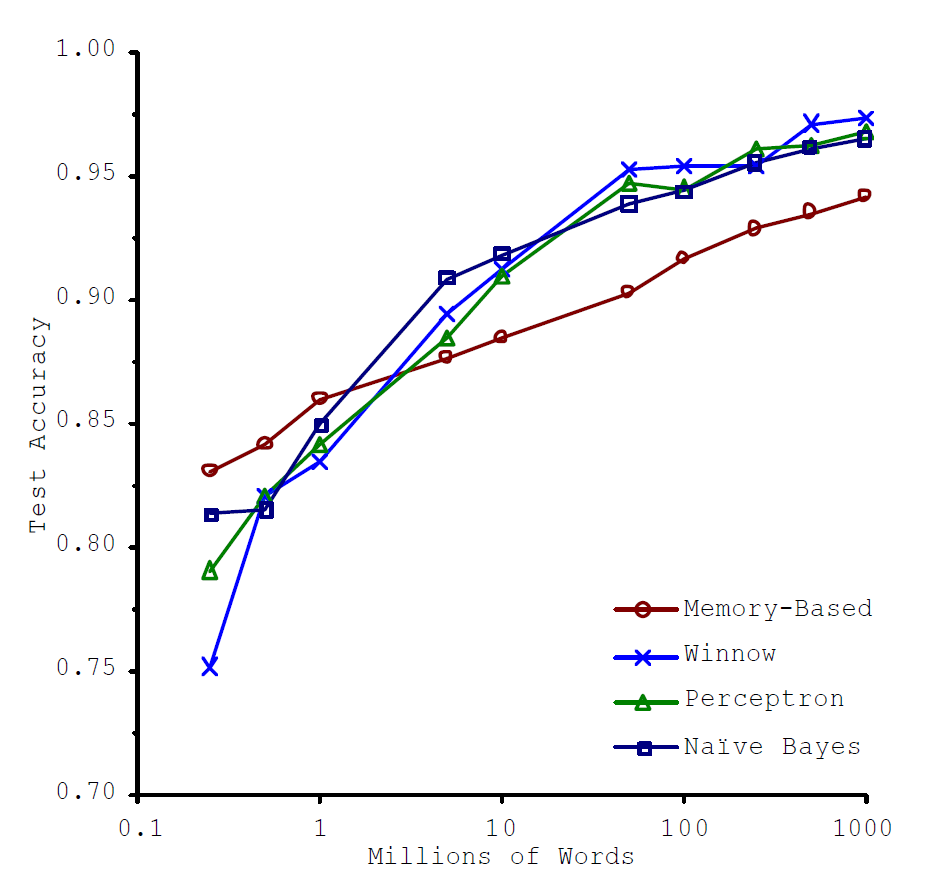
What is the link between machine learning and big data? Here a quote from Andrew Ng about machine learning (Ng, 2015): “it’s not who has the best algorithm that wins. it’s who has the most data.” This claim has been empirically corroborated by the work of Banko and Brill (“Scaling to Very Very Large Corpora for Natural Language Disambiguation,” 2001) from Microsoft Research; they present a study of the effects of data size on machine learning for natural language disambiguation. Figure 3 illustrates without ambiguity the conclusion of that study—this graph is extracted from the paper of Banko and Brill (“Scaling to Very Very Large Corpora for Natural Language Disambiguation,” 2001).
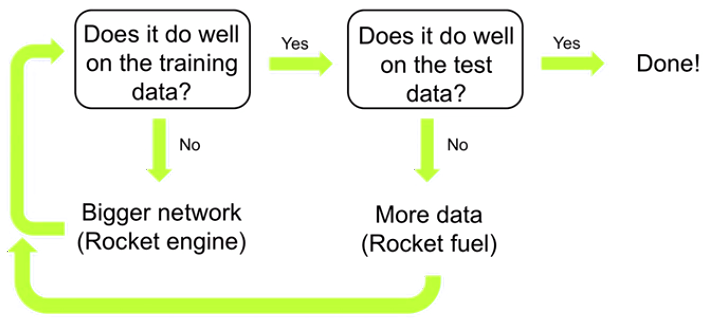
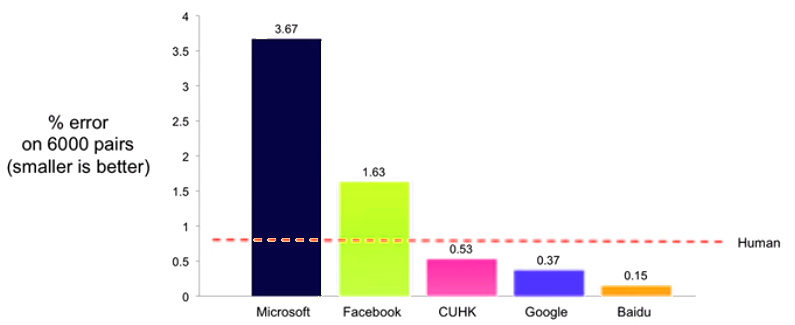
Ng is one of the most renowned scientists in the realm of machine learning. He is the initiator of the ambitious Google Brain project—and he led that project for several years (Wikipedia, 2015)—he is teaching machine learning at Stanford University (his course is meeting extreme popularity with many hundred students enrolling every year—he also teaches Machine Learning on Coursera, a MOOC platform he co-founded), and recently he has joined Baidu for building even more powerful machine learning-based technologies. Earlier this year, he gave an interesting talk at the “GPU Technology Conference 2015” (the video can be watched at ustream.tv). During the conference he made a very comprehensive metaphor. He compared machine learning with space rockets. To launch a many tones rocket in orbit, you need both a very powerful engine and a massive quantity of fuel. If you have a big engine and only a little fuel, the rocket will not even take off the ground. Conversely, if you inject an inordinate quantity of fuel in an undersized engine, the rocket will, in all likelihood, explode immediately after ignition. The fuel is the big data, whereas the engine is the machine learning technology, which consists of both smart algorithms and a substantial computing power. Figure 4 depicts the general approach to develop a machine learning solution; it suggests that the need for data can be boundless in order to reach an adequate outcome. Big data and machine learning are synergistically spiraling up. One needs the other to be harnessed to its full potential; and that potential seems growing real fast. Figure 5 shows a rather unsettling result; computers now outperform humans in face recognition, an activity in which the human cognitive system is supposed to excel.
Knowing Customers
How much a company that successfully harnesses the combo big data/machine learning can manage to know about its customers? Well, it obviously depends on the company, but in general a lot more than most people think. Some of the large retailers (e.g., Amazon, Tesco, Target) have already successfully applied basic techniques to leverage their data in an effort of implementing a customer centricity approach. The New York Times published a comprehensive article that gives some flavors of what is currently done (Duhigg, 2012).
Target achieved worldwide notoriety for their effective pregnancy predictor. The method very accurately determines whether a woman is pregnant based on her shopping attitude and, if she is, yields an astonishingly precise estimation of the due date. Their model (and motivation1) reposes on the recent findings on habits, a field that has gained traction in recent years (Duhigg, 2012).
Amazon envisages shipping items even before the customer orders them. This pioneering concept denotes how confident Amazon is in knowing its shoppers. The fact that they claimed a patent for this idea suggests that Amazon believes such practices might become common (i.e., the idea prevails over the technological prowess). The title of the patent says it all: Method and System for Anticipatory Package Shipping (Spiegel et al., 2013).
From an airline perspective, being able to reliably anticipate the travel needs for its customers (existing or potential) is certainly a major trump card, and would significantly contribute in reckoning the lifetime value of a customer.
Air tickets are now commonly purchased online, and represent a significant share of the total online spending (Harteveldt, 2012); and as Warner (How Predictive Analytics Elevate Airlines’ Customer Centricity and Competitive Advantage, 2011) shrewdly observes, online retailers and airlines are alike in many respects; in particular, their customers “are influenced by many of the same factors,” (How Predictive Analytics Elevate Airlines’ Customer Centricity and Competitive Advantage, 2011, p. 1) making the cases discussed above even more pertinent.
Capturing Relevant Data
“Annual global IP traffic will surpass the zettabyte2 (1000 exabytes) threshold in 2016},” according to Cisco (Cisco Visual Networking Index: Forecast and Methodology, 2013–2018, 2014, p. 1). The “big” in “big data” delivers all its semantic significance here, and appears well deserved. The traditional old Internet of the last century still savagely growing, the new and steep increase in the adoption of social media in daily life, the rapid proliferation of ubiquitous computing (as predicted by Xerox some decades ago (Weiser, 1999)—recently dubbed the “Internet of Things,” and often written IoT) all contribute to the creation and collection of various data, both structured and unstructured3—but mostly unstructured (Holzinger et al., 2013). How to amass even a fraction of that? And even more importantly, how to get the relevant bit?
As noted earlier, airlines pioneered eCommerce. The wisest of them must have been storing plenty of valuable information. Furthermore, the IATA-commissioned report (Paul et al., 2013) reveals that the online spending from business and leisure travelers for airlines should reach $110.2 billion in 2016 (an estimation made in 2012, although newer estimations might be more accurate, they likely remain in the same order of magnitude), laying the ground for numerous opportunities to harvest many silos of data.
Nevertheless, all the relevant data are not readily available to airlines. Search engines (i.e., Google or Baidu), social media (e.g., Facebook and the like) or travel-related websites (e.g., Booking.com, TripAdvisor, TripIt and other comparable services) are getting large and interesting pieces of the puzzle. The IATA report recommends to carefully watch what it coins the CAFGA, the “gang of five” consisting of Concur, Apple, Facebook, Google, and Amazon. Those titans of the Internet might eventually play a major role in the airline industry, especially as data providers (in exchange for money). The bid-ask game for data trading is about to get mercilessly intense.
Meanwhile, confidentiality and privacy is a serious matter (Executive Office of the President, 2014), and the necessary race to data acquisition takes a rather thorny path, and the thorns might be of unequal length for the different actors (e.g., depending on local legislation or culture). Overall, public opinion/perception is not to be considered lightly (Afroz et al., 2013; Rust et al., 2002). The social media era has empowered Internet users. Although such media are providing brands with a powerful means of promoting themselves, a single slip-up can damage, or even ruin, the reputation of a brand. Users are more and more concerned with privacy, and are aware of the potential value of their own personal data. The uncertainty about the possible drift and subsequent consequences in the future has become a source of worry to many users, making them extra-cautious. Many of the major Internet players are often under scrutiny for their handling of data, and are to respond to various polemics and controversies. A corporation suspected of misconduct regarding privacy will likely have to face the wrath of the crowd.
Although predictions vary, the attitude of users regarding privacy and data sharing may evolve toward more tolerance (Rainie & Anderson, 2014).
Panacea for Traditional Airlines?
The fact that many traditional airlines are struggling to remain competitive, in spite of an already huge, nonetheless still rapidly growing market, is difficult to ignore.
Many of the referenced materials suggest that predictive analytics cannot be overlooked, and is described as a necessary step toward success (Brown et al., 2011). The science is quite solid and the early adopters have demonstrated very encouraging results, which should convince even an empirical skeptic that such an approach has the potential to boost businesses.
Having said that, it is prudent to bear in mind that some of the institutions/firms that sponsored the writing of certain of those cited documents have some interest in selling solutions or services (such as IBM or McKinsey). They might conveniently set the focus on the potential benefits without over-insisting on the multiple challenges that must be overcome to implement the method in an already mature and rather conservative environment.
Besides, successfully implementing a data-driven strategy does not mean being shielded against poor decisions. Tesco was a splendid example of successfully leveraging data to increase value, until a couple of years ago. The recent figures showing that the retailer is alarmingly in decline has raised some serious questions about the supremacy promised by predictive analytics (Schrage, 2014).
In my view, the combo big data/machine learning constitutes one of the best means of successfully implementing a customer centricity approach and the airline industry is prone to benefit from such an approach. Is the goal overambitious and the technology too premature? It is ambitious, indeed, but within reach provided a deep commitment, both in terms of management and in terms of resources. The technology is already there, and exponentially improving. ■
(Cover photo by Artur Tumasjan on Unsplash.com)
References
- Afroz, S., Islam, A. C., Santell, J., Chapin, A., & Greenstadt, R. (2013). How privacy flaws affect consumer perception. Workshop on Socio-Technical Aspects in Security and Trust, STAST, 10–17. https://doi.org/10.1109/STAST.2013.13
- Banko, M., & Brill, E. (2001). Scaling to Very Very Large Corpora for Natural Language Disambiguation. ACL ’01 Proceedings of the 39th Annual Meeting on Association for Computational Linguistics, 26–33. https://doi.org/10.3115/1073012.1073017
- Barbot, C., Costa, Á., & Sochirca, E. (2008). Airlines performance in the new market context: A comparative productivity and efficiency analysis. Journal of Air Transport Management, 14(5), 270–274.
- Bilotkach, V., Gaggero, A. A., & Piga, C. A. G. (2011). Airline Pricing under Different Market Conditions: Evidence from European Low-Cost Carriers. Working Paper Series 47-11, The Rimini Centre for Economic Analysis. https://doi.org/10.1016/j.tourman.2014.09.015
- Bishop, C. (2006). Pattern Recognition and Machine Learning. Springer.
- Blyton, P., Lucio, M. M., McGurk, J., & Turnbull, P. (2001). Globalization and trade union strategy: Industrial restructuring and human resource management in the international civil aviation industry. International Journal of Human Resource Management, 12(3), 445–463.
- Brown, B., Chui, M., & Manyika, J. (2011). Are you ready for the era of ’big data’? In McKinsey Quarterly: Vol. October (October; Number October, pp. 1–12). https://goo.gl/VRQzkY
- Budd, L., Francis, G., Humphreys, I., & Ison, S. (2014). Grounded: Characterising the market exit of european low cost airlines. Journal of Air Transport Management, 34, 78–85. https://doi.org/10.1016/j.jairtraman.2013.08.002
- CODA. (2013). Delays to Air Transport in Europe - Annual 2013. Central Office for Delay Analysis (CODA).
- Cisco. (2014). Cisco Visual Networking Index: Forecast and Methodology, 2013–2018. https://goo.gl/JTaslI
- Dresner, M., Eroglu, C., Hofer, C., Mendez, F., & Tan, K. (2015). The impact of Gulf carrier competition on U.S. airlines. Transportation Research Part A: Policy and Practice. https://doi.org/10.1016/j.tra.2015.03.022
- Dresner, M., Lin, J.-S. C., & Windle, R. (1996). The Impact of Low-Cost Carriers on Airport and Route Competition. Journal of Transport Economics and Policy, 30(3).
- Duhigg, C. (2012). How Companies Learn Your Secrets. The New York Times. https://goo.gl/McyltL
- Fader, P. (2012). Customer Centricity. Wharton Digital Press. https://goo.gl/LWnN68
- Harteveldt, H. H. (2012). The Future Of Airline Distribution - A Look Ahead To 2017 (pp. 2–32). Special Report Commissioned by IATA. https://goo.gl/0jMTX6
- Holzinger, A., Stocker, C., Ofner, B., Prohaska, G., Brabenetz, A., & Hofmann-Wellenhof, R. (2013). Combining HCI, natural language processing, and knowledge discovery - Potential of IBM content analytics as an assistive technology in the biomedical field. Lecture Notes in Computer Science (Including Subseries Lecture Notes in Artificial Intelligence and Lecture Notes in Bioinformatics), 7947 LNCS, 13–24. https://doi.org/10.1007/978-3-642-39146-0_2
- Hätty, H., & Hollmeier, S. (2003). Airline strategy in the 2001/2002 crisis—the Lufthansa example. Journal of Air Transport Management, 9(1), 51–55.
- Keiningham, T. L., Aksoy, L., Cooil, B., & Andreassen, T. W. (2012). Linking customer loyalty to growth. MIT Sloan Management Review, 1. https://goo.gl/tzzbQI
- Kurzweil, R. (2011). IT growth and global change: A conversation with Ray Kurzweil. https://goo.gl/XU8SbX
- Moosberger, M., & Pearce, B. (2007). Economics Briefing—Airline Cost Performance. IATA. https://goo.gl/4d8pOF
- Ng, A. (2015). Lecture notes in Machine Learning. https://goo.gl/LWUAIw
- Paul, A., Menon, S. U., & Iyangar, J. (2013). Customer centricity in airline industry. Mindtree. https://goo.gl/Dd8Nmz
- Pilz, S., & Dyerfox, J. (2011). Capturing value from customer-centric sales and services. IBM Center for Applied Insights. https://goo.gl/FvH6hI
- Power, B. (2015). Artificial Intelligence Is Almost Ready for Business. Harvard Business Review. https://goo.gl/bYDaVs
- Rainie, L., & Anderson, J. (2014). The Future of Privacy. Pew Research Center. https://goo.gl/qgVhTp
- Rust, R. T., Kannan, P. K., & Peng, N. (2002). The Customer Economics of Internet Privacy. In Journal of the Academy of Marketing Science (Vol. 30, Number 4, pp. 455–464). https://doi.org/10.1177/009207002236917
- Schmidt, E., & Rosenberg, J. (2014). How google works. Hachette UK.
- Schrage, M. (2014). Tesco’s Downfall Is a Warning to Data-Driven Retailers. Harvard Business Review. https://goo.gl/EQ1VoL
- Sherry, L. (2015). A method for quantifying travel productivity for corporate travel managers. Journal of Air Transport Management, 42, 118–124. https://doi.org/10.1016/j.jairtraman.2014.09.012
- Smyth, M., & Pearce, B. (2006). Airline Cost Performance—IATA Economics Briefing N. 5. IATA. https://goo.gl/dE8E5B
- Spiegel, J. R., McKenna, M. T., Lakshman, G. S., & Nordstrom, P. G. (2013). Method and system for anticipatory package shipping. Google Patents. https://goo.gl/K0u4a4
- Treacy, M., & Wiersema, F. D. (1993). Customer intimacy and other value disciplines. Harvard Business Review, 71, 84–93. https://goo.gl/7gwXw4
- Treacy, M., & Wiersema, F. D. (1997). The Discipline of Market Leaders: Choose Your Customers, Narrow Your Focus, Dominate Your Market. Basic Books.
- Tretheway, M., & Andriulaitis, R. (2015). What Do We Mean by a Level Playing Field in International Aviation? International Transport Forum.
- Warner, S. (2011). How Predictive Analytics Elevate Airlines’ Customer Centricity and Competitive Advantage. Cognizant. https://goo.gl/vrV6Eq
- Weiser, M. (1999). The computer for the 21st century. Mobile Computing and Communications Review, 3(3), 3–11. https://doi.org/10.1145/329124.329126
- Wikipedia. (2015). Sabre (computer system)—Wikipedia, The Free Encyclopedia. https://goo.gl/uTQIDs
- Wikipedia. (2015). Deep learning—Wikipedia, The Free Encyclopedia. https://goo.gl/wfDLvU
- Wikipedia. (2015). Google Brain—Wikipedia, The Free Encyclopedia. https://goo.gl/w8hv2G
- De Mauro, A., Greco, M., & Grimaldi, M. (2015). What is big data? A consensual definition and a review of key research topics. AIP Conf. Proc., 97–104. https://doi.org/10.1063/1.4907823
- Executive Office of the President. (2014). Big Data: Seizing Opportunities, Preserving Values. https://goo.gl/Ppx44Y
-
Understanding and manipulating habits may prove very efficient in customer retention. ↩
-
1 ZB = 1021 bytes. ↩
-
Here again a concept widely uttered, but which ultimately lacks a formal definition. The fine line that separates structured and unstructured data is often blur, requiring subjectivity to decide when getting in the vicinity of the border. ↩